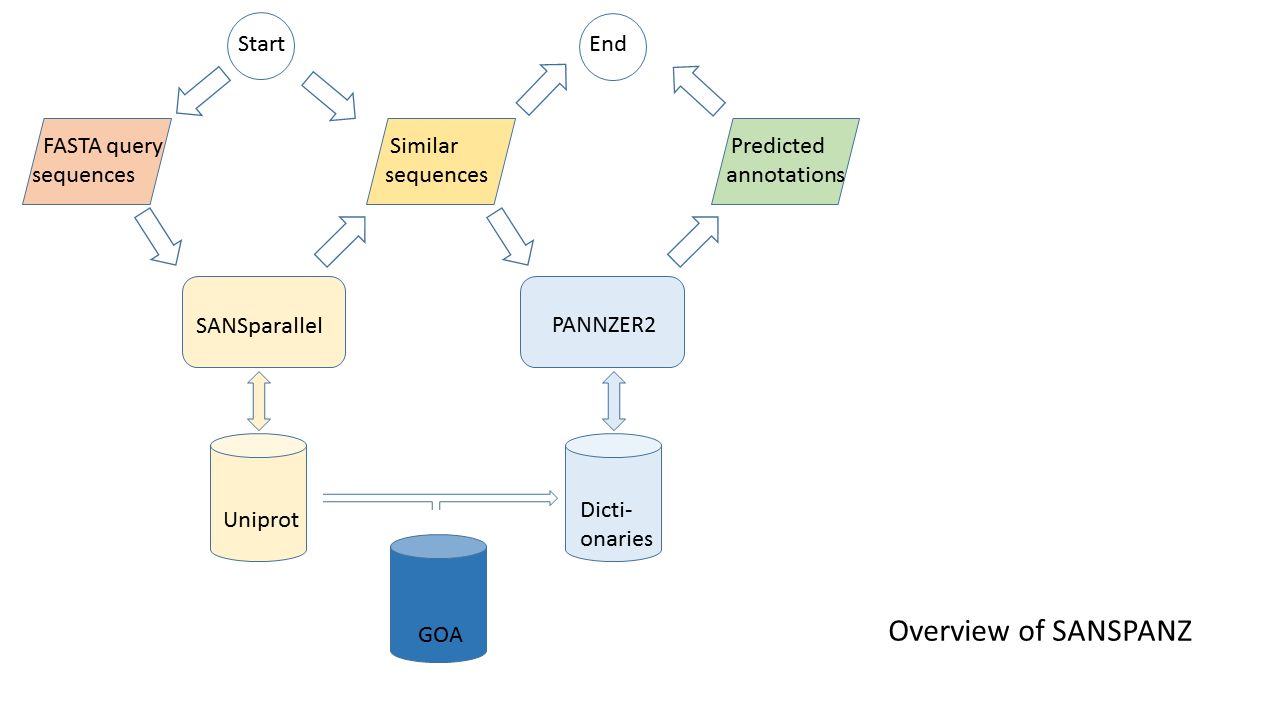
Figure 1: Overview of SANSPANZ (combination of SANSparallel and PANNZER).
The web server is the recommended way of using PANNZER. Standalone scripts (Download tab) are provided for the sake of transparency.
Pannzer2 (this version) is a thousand times faster and more user-friendly than Pannzer1.
Interface | Ease of use | Speed |
---|
Pannzer2 web server | ++ | +(+) |
Pannzer2 standalone with remote databases | + | + |
Pannzer2 standalone with local databases | - | ++ |
Pannzer1 standalone | -- | -- |
Pannzer1 at CSC (discontinued) | + | - |
Pannzer2 at CSC (integrated to Chipster) | + | + |
Web server
The query form is self-explanatory. Inputs are query sequences in FASTA format, the scientific name of the organism from which the query sequences come, and optionally an email address for notification when a batch job finishes.
The scientific name is used by the RM3 scoring function to calculate taxonomic distances. If the field is left blank, PANNZER2 will automatically substitute a good guess of the query species.
The web server's submission form is under the
Annotate tab. Precomputed example results can be browsed under the
Examples tab.
The query form has two options with slightly different outputs. Interactive jobs are limited to ten sequences and results are shown for the Argot predictor, which uses an information content weighted sum.
Batch jobs are limited to 100,000 sequences. The resul page shows the submission and start time, how many query sequences have been processed, and time at finish. Toggle parameters to change predictors from Argot (default) to RM3 (a regression model similar to the original Pannzer article), Blast2GO, hypergeometric (HYGE) or Jaccard (JAC). If the query sequences are fragments, it can be beneficial to change the sequence filtering to accept hits that fulfil the coverage criterion for query OR sbjct (the default is AND). It the query sequences are short fragments, it can be beneficial to lower the minimum alignment length from 100 amino acids. The HTML summary is paginated to report 1000 queries per file. The HTML pages are meant for browsing.
The table has columns for gene symbol (GN), description (DE), three Gene Ontologies (GO) and an inverse mapping from the highest scoring GO prediction to enzyme classes (EC) or pathways (KEGG).
Text outputs are available for downloading.
The downloadable file named "GO prediction details" and "DE prediction details" contain intermediate results for each query. The final results are in the "annotations" file. It is a parseable file, which uses a context sensitive grammar, as explained below. The file has six tab-separated columns labelled qpid, type, score, PPV, id and desc. The first column (qpid) always contains the identifier of the query sequence. The second column (type) can take the following values, and the score, PPV, id and desc columns change meaning accordingly:
- original_DE: score is euk for eukaryotic query species and bac otherwise; id is the form factor of desc, the description from the input FASTA file
- qseq: desc is the amino acid sequence of the query protein
- DE: score is the prediction score; PPV is the normalized prediction score between 0 and 1; id is the form factor of desc, the predicted description
- GN: desc is the gene symbol. A gene symbol is predicted if its support is greater than half in the list of homologs
- ontology_predictor where ontology is one of MF (molecular function), BP (biological process), CC (cellular component) and predictor is one of RM3, ARGOT, HYGE or JAC. score is the prediction score, PPV is the normalized prediction score between 0 and 1, id is the GO identifier and desc is the short description of the GO class
- EC_predictor: desc is the GO class that has the highest PPV and has a link in ec2go, id is the EC class
- KEGG_predictor: desc is the GO class that has the highest PPV and has a link in kegg2go, id is the KEGG pathway identifier
Frequently asked questions
What to do with RNA-seq data?
For example, assemble transcripts using Trinity, predict peptides using TransDecoder or
ORFfinder, and submit FASTA-file of predicted peptide sequences to Pannzer2.
Which PPV threshold should I use?
This discussion uses ARGOT scores as example, but the principles are the same for other scores as well.
ARGOT_score is the result from the internal function of PANNZER, which quantifies how strongly overrepresented a given GO class is. We have currently no simple rule for interpreting this value. Therefore we generated the PPV estimate below.
PPV (Positive Predictive Value) is an estimate for the reliability of the predicted GO class. It estimates the reliability of the GO class being correct or approximately correct. Approximately correct here means that the correct GO class is near the predicted GO class in the GO tree (a child or parent node of the correct GO class). The relationship between PPV and Argot score was calibrated using a training set of proteins for which the correct annotation was known.
PPV = Good_Stuff/(Good_Stuff + Bad_Stuff)
Good_Stuff is the number of correct or approximately correct predictions with the approximately same Argot Score.
Bad_Stuff is the number of clearly bad predictions with approximately the same Argot Score.
We have intentionally not given exact clear rules how the PPV values should be used. We have more reliable and less reliable predictions in PANNZER results. The following are recommendations:
- Download the PANNZER results with the PPV scores.
- Generate a list of threshold values (examples: [0.9, 0.8, 0.7, 0.6, 0.5], [0.8, 0.6, 0.4])
- Generate a few separate sets of PANNZER results, each filtered with the selected threshold value.
Keep only the results that have a larger PPV than the threshold in the results.
- Do some preliminary downstream analysis with each separate set.
- Select a set (or sets) that look good in preliminary analysis for further steps.
Data security
Uploaded sequence data and results are deleted after one week. The URL to the results is chosen randomly from a trilliion alternatives. Those concerned about security should download SANSPANZ and run the script locally. The SANSparallel server, used for homology searches, does not store any user data on disk.
User email addresses and job names are logged for notification purposes.
Stand-alone script
The script is available from the
Download tab. Inputs for the examples of running runsanspanz.py below are in the testdata/ subdirectory of the distribution package and outputs can be found in the testresults/ subdirectory.
Simple usage with remote databases
The distribution package includes a simple script that can perform specific tasks. Help text is printed when the script is run without arguments. The script accesses remote sequence search and GO database servers, so no databases or servers need to be installed locally. The most frequently used command-line options are listed below.
Option | argument | default |
-f | input format, either FASTA or tab | FASTA |
-i | input file | STDIN |
-m | method | Pannzer |
-o | output files (csv) | STDOUT,method.out_1,method.out_2,... |
-s | species | automatically parsed from Uniprot style header |
-R | [a flag to send server requests to remote servers] | use local servers |
This example uses default options for functional annotation of macaque proteins using Pannzer:
python runsanspanz.py -R -s "Macaca mulatta" < testdata/querysequences.fasta
perl anno2html.pl < Pannzer.out_3 > predictions.html
The output is written to STDOUT and files named Pannzer.out_1, Pannzer.out_2, Pannzer.out_3. Pannzer.out_1 contains details of the description (DE) prediction. Pannzer.out_2 contains details of the GO prediction. Pannzer.out_3 is a summary of all predicted annotations, which is converted to HTML by the anno2html.pl script.
Here, we direct outputs to files with descriptive names:
python runsanspanz.py -R -m Pannzer -s "Macaca mulatta" -i testdata/querysequences.fasta -o ",DE.out,GO.out,anno.out"
You can store the sequence search result from SANSparallel in a tabular format for later analysis. Below, the second command runs Pannzer using inputs in tabular format.
python runsanspanz.py -R -m SANS -s "Macaca mulatta" -i testdata/querysequences.fasta -o sans.tab
python runsanspanz.py -R -m Pannzer -i sans.tab -f tab -o ",DE.out1,GO.out1,anno.out1"
Pannzer2 reports predictions for descriptions (DE) and for GO terms using four predictors (RM3,ARGOT,JAC,HYGE). You can restrict the number of predictors used. ARGOT has been one the top predictors in our benchmarks.
python runsanspanz.py -R -m Pannzer -i sans.tab -f tab -o ",DE.out2,GO.out2,anno.out2" --PANZ_PREDICTOR "DE,ARGOT"
Pannzer2 applies strict filtering criteria to the sequence neighborhood, including default query and sbjct coverage of 70 %. The flag --PANZ_FILTER_PERMISSIVE relaxes the coverage criteria so that 70 % coverage is required of query or sbjct, but not both. This can result in more sequences getting predicted annotations (at the expense of accuracy).
python runsanspanz.py -R --PANZ_FILTER_PERMISSIVE -m Pannzer -i sans.tab -f tab -o ",DE.out3,GO.out3,anno.out3"
BLAST is more sensitive than SANSparallel at detecting distantly related proteins, but these are removed by Pannzer2 when filtering the sequence neighborhood, and
BLAST is orders of magnitude slower than SANSparallel. If anyone desperately wants to use BLAST, we provide a script that converts BLAST output to our tabular format. The database must be Uniprot. Edit the variable $BLAST_EXE on line 11 of runblast.pl to correspond to your local environment.
perl runblast.pl testdata/querysequences.fasta testdata/uniprot.fasta 40 > blast.tab
python runsanspanz.py -R -m BestInformativeHit -i testdata/blast.tab -f tab -o ',--' 2> err
python runsanspanz.py -R -m Pannzer -i testdata/blast.tab -f tab -o ',DE.out4,GO.out4,anno.out4'
Pannzer expects protein sequences as input. We provide a utility script that extracts ORFs (longer than 80 aa) from nucleotide sequences:
perl longestorf.pl 80 < nucleotidesequences.fa > orfs.fasta
python runsanspanz.py -R -m Pannzer -i orfs.fasta -f FASTA -o --PANZ_PREDICTOR ARGOT ',,,orfs.anno'
Parameter configuration
Parameters can be changed using command line options, a configuration file, or within a Python script.
Command line options are shown by
python runsanspanz.py -h
A
configuration file can be specified on the command line:
python runsanspanz.py -a myparameters.cfg
The configuration file need only define values for a subset of parameters. Other parameters will remain at their default values.
The current configuration can be written out to a file for later reuse or editing:
import Parameters
glob=Parameters.WorkSpace()
glob.writeConfigFile('myparameters.cfg')
Local database servers
SANSPANZ predicts functional descriptions and Gene Ontology (GO) terms using summary statistics calculated from the sequence neighborhood of the query sequence and database background frequencies.
This leads to the complex architecture and web of dependencies depicted in Figure 1. There are two client-server interactions. SANSPANZ is a client sending requests to the SANSparallel and DictServer servers.
Server | Purpose | Parameters |
SANSPANZ/DictServer.py [OPTIONS] | Server providing database statistics and GO associations when SANSPANZ is run locally. | CONN_HOSTNAME, CONN_PORTNO |
SANSparallel server | Server providing sequence similarity search results when SANSPANZ is run locally. | CONN_SANSHOST, CONN_SANSPORT |
We run the servers on localhost at port 54321 and 50002, respectively. The servers are started as follows:
module add openmpi-x86_64
nohup mpirun -np 17 -output-filename /data/uniprot/u SANSparallel.2/server /data/uniprot/uniprot 54321 uniprot.Dec2015 &
nohup python ./SANSPANZ/DictServer.py --CONN_PORTNO 50002 &
Updating local databases
It is imperative that DictServer tables refer to the same database release that is searched by SANSparallel. Therefore, use both remote SANSparallel and DictServer servers, or install both locally.
The following update procedure assumes that the local databases reside in /data/uniprot. It uses runsanspanz.py and DictServer.py from SANSPANZ and the following utility scripts from the ./uniprot/ subdirectory: external2go.pl, sanspanz_cleaner.py, sumcol.pl, generate_godict.pl and program gorelatives.
# taxonomy
curl "https://www.uniprot.org:443/taxonomy/?query=*&compress=yes&format=tab" > taxonomy-all.tab.gz
rm -f taxonomy-all.tab
gunzip taxonomy-all.tab.gz
# EC and KEGG mappings to GO
rm -f ec2go kegg2go
wget http://geneontology.org/external2go/ec2go
perl external2go.pl < ec2go > ec2go.tab
wget http://geneontology.org/external2go/kegg2go
perl external2go.pl < kegg2go > kegg2go.tab
# update counts: uniprot.phr is indexed by SANSparallel and contains the description lines of the SANSparallel sequence database
perl -pe 's/^\S+\s+//' /data/uniprot//uniprot.phr| perl -pe 's/ \w+\=.*//' | python runsanspanz.py -m Cleandesc -f tab -c desc -b desc | cut -f 2 > x
sort x | uniq -c > uniprot.desc.uc.counts
perl -pe 's/ +/\n/g' x | sort | uniq -c > uniprot.word.uc.counts
perl sumcol.pl 1 < uniprot.desc.uc.counts > nprot
perl sumcol.pl 1 < uniprot.word.uc.counts > nwordtotal
# GO dictionaries
rm -f goa_uniprot_all.gaf
wget ftp://ftp.ebi.ac.uk/pub/databases/GO/goa/UNIPROT/goa_uniprot_all.gaf.gz
gunzip goa_uniprot_all.gaf.gz
rm -f go-basic.obo
wget http://geneontology.org/ontology/go-basic.obo
# GO dictionaries using gorelatives
# GO hierarchy and information content of GO terms
python runsanspanz.py -m obo -i /data/uniprot/go-basic.obo -o 'obo.tab,'
cut -f 2,5,7 /data/uniprot/goa_uniprot_all.gaf | python runsanspanz.py -m gaf2propagated_with_evidencecode -f tab -c "qpid goid evidence_code" -o ",/data/uniprot/godict.txt" 2> err
cut -f 2 /data/uniprot/godict.txt | sort -T /data/uniprot | uniq -c | perl -pe 's/^\s+//' | perl -pe 's/ /\t/' > godict_counts
python runsanspanz.py -m BayesIC -i godict_counts -f tab -c "qpid propagated" --eval_OBOTAB obo.tab -o ",obo_with_ic.tab"
# GO propagated parent list using gorelatives
grep 'id: GO:' /data/uniprot/go-basic.obo | perl -pe 's/\S*id: GO://' > /data/uniprot/go.list
./gorelatives -b go-basic.obo -q /data/uniprot/go.list -r isa,partof,altid -d parents -l > /data/uniprot/go_data
perl generate_godict.pl /data/uniprot/go_data obo_with_ic.tab ec2go.tab kegg2go.tab > /data/uniprot/mergeGO.out
# restart DictServer for SANS-PANZ:
python DictServer.py --CONN_PORTNO 50002 &
DictServer reads mergeGO.out (glob.param['DATA_GOIDELIC']) and godict.txt (glob.param['DATA_GODICT']).
The species list is consulted by a CGI script, which is part of the web server, to give hints of the scientific names matching the prefix entered by the user:
cut -f 3 /data/uniprot/taxonomy-all.tab > specieslist
Evaluation of GO predictions
The runsanspanz.py script can be used to evaluate the correctness of GO predictions using similar metrics as in the CAFA competition. The evaluation metrics are
(1) F = 2 * pr * re / (pr + re) = 2 * TP / (T + P)
(2) J = TP / (T + P - TP)
(3) S = 1/ne * sqrt(mi^2 + ru^2) = 1/ne * sqrt( (wP-wTP)^2 + (wT-wTP)^2 )
(4) wF = 2 * wTP / (wT + wP)
(5) wJ = wTP / (wT + wP -wTP)
where pr is precision, re is recall, ru is remaining uncertainty and mi is misinformation. T, P and TP refer to [the cardinality of sets of] nodes in the GO hierarchy. T are the GO terms associated with a query protein in the reference of truth. P are the predicted GO terms above a score threshold tau. TP are the intersection of T and P ("true positives"). T, P and TP give a unit weight to each node. wT, wP and wTP weigh the nodes by their information content conditional on the parent nodes in the GO hierarchy being also true/predicted (
Clark and Radivojac 2013).
Fmax, wFmax, Jmax, wJmax and Smin are the optimum value reached at any score threshold. Smin is normalized by the number of proteins with predictions, ne.
Predicted GO annotations are compared to a reference of truth. Below, the truth is extracted from GOA file based on experimental evidence. Your actual test set is probably a subset of this.
cut -f 2,5,7 /data/uniprot/goa_uniprot_all.gaf | egrep 'EXP|IDA|IPI|IMP|IGI|IEP' | python runsanspanz.py -R -m gaf2propagated -f tab -c "qpid goid evidenceCode" -o ",goa_truth_propagated"
Generate predictions using the Pannzer method. Assuming that predictions and the reference of truth have matching protein accession numbers, evaluation metrics are output by the GOevaluation method:
python runsanspanz.py -R -m gaf2propagated -f tab -i testdata/gotest_truth.gaf -o ",gotest_truth_propagated" -c "qpid goid"
python runsanspanz.py -R -m GOevaluation -f tab -o ',,eval1' -i testdata/GO.out --eval_SCOREFUNCTIONS "RM3_PPV ARGOT_PPV JAC_PPV HYGE_PPV" --eval_TRUTH testdata/gotest_truth_propagated
As in CAFA, we provide a naive method uses the base frrequency of GO terms as the probability of prediction:
python runsanspanz.py -R -m naive -i testdata/target_identifiers.list -o ',naive_predictions.out' -c 'qpid' -f tab
python runsanspanz.py -R -m GOevaluation -i testdata/naive_predictions.out -f tab -o ',,eval2' --eval_SCOREFUNCTIONS "frequency" --eval_TRUTH testdata/gotest_truth_propagated 2> err
paste eval1 eval2
Evaluation of DE predictions
DSM is a description similarity measure which is the cosine similarity of TF-IDF weighted word vectors. It can be used to compare DE predictions by Pannzer to a reference of truth (e.g., the original descriotions). The following example first runs Pannzer to generate DE predictions, prepares a reference of truth, and finally runs the evaluation:
python runsanspanz.py -R -m Pannzer --PANZ_PREDICTOR DE -i human_subset.fasta -f FASTA -o 'human_subset.sans,DE.out,,'
grep '^>' human_subset.fasta| perl -pe 's/ /\t/' | perl -pe 's/^>//' | python runsanspanz.py -R -m wordweights -c "qpid desc" -f tab -k 1000 > human_subset_de.tab
python runsanspanz.py -R -m DE_evaluation -i DE.out -f tab --eval_TRUTH human_subset_de.tab -o ",DE.eval"
Customization
Pannzer2 is implemented in the
SANSPANZ framework. New functionalities are easily implemented by creating new operator classes.